NSF AI Institute For Research On Trustworthy AI In Weather, Climate, and Coastal Oceanography (AI2ES)
Sponsor: National Science Foundation
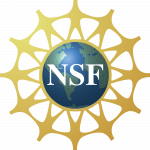
Collaborators
LEAD INSTITUTION
The University of Oklahoma
ACADEMIC PARTNERS
Colorado State University
Del Mar College (TX)
North Carolina State University
State University of New York at Albany
Texas A&M University - Corpus Christi
University of Washington
INDUSTRY PARTNERS
IBM and The Weather Company
Disaster Tech
Nvidia
FEDERALLY FUNDED RESEARCH LABS AND FEDERAL PARTNERS
NCAR / UCAR
NOAA
Funding Period
September 2020 – August 2025
Description
AI2ES VISION
The vision of AI2ES is to create trustworthy Artificial Intelligence (AI) methods for diverse environmental science users that will revolutionize our understanding and prediction of high-impact atmospheric and ocean science phenomena and create new educational pathways to develop a more diverse AI and environmental science workforce.
THE NEED FOR AI2ES
Changes in weather patterns, oceans, sea level rise, and disaster risk amplify the need for accelerated AI research in the environmental sciences. AI2ES is a convergent, multi-sector NSF Trustworthy AI institute led by the University of Oklahoma that brings together researchers in AI, atmospheric science, ocean science, and risk communication.
NCSU EFFORT
The NCSU team will conduct coastal ocean research in three areas:
1) User-inspired AI/ML research on ocean environment / Coastal Flooding
Floods are one of the most common and destructive natural disasters. They cause massive damage to human life, infrastructure, and socioeconomic system. Floods can occur due to heavy rains, overflowing rivers, storm surges, and tsunamis, melting of ice caps and glaciers, etc. Over the recent years, climate change has increased the intensity of occurrence of coastal flooding mainly through sea-level rise and storm surge. Accurate coastal flood predictions are therefore important for hazard assessment and extreme event management. Several recent studies have shown that changes in the joint distributions of storm surge and precipitation associated with climate variability and change augment flood potential in low-lying coastal areas. Knowing the probability of these compound events and understanding the processes driving them is essential to predict and mitigate the associated high-impact flooding risks. We will advance existing coastal flood prediction at different time scales by developing new physically-based AI/ML methods that consider all environmental factors such as water level, winds, soil moisture, river discharge, precipitation, ground temperature, etc. to address increasing risk of compound flooding from storm surge and rainfall.
2) User-inspired AI/ML research on ocean environment / Ocean Eddies
The ocean, like the atmosphere, is a fundamentally turbulent system. As a consequence, intense nonlinear interactions that give origin to small scale structures such as eddies, fronts, jets and filaments, dominate the ocean circulation. Mesoscale eddies are ubiquitous in the ocean, and typically exhibit different characteristics to their surroundings, allowing them to transport properties such as heat, salt and carbon around the ocean. This takes place everywhere in the world’s ocean and at all latitude bands. Most of the mesoscale eddy energy is generated by instabilities of the mean flow, and by air-sea interactions. They can feed energy and momentum back into the mean flow and help drive the deep ocean circulation and they are suspected to be the key element in transporting and mixing water properties as they propagate in the ocean, playing a significant role in the global budgets of ocean tracers and climate. They have also a major impact on ecosystems, and society by affecting fisheries, offshore platforms etc. In this project, we will focus on the Loop current eddy (LCE), which is the dominant circulation process in Gulf of Mexico waters. As the LC meanders from the Yucatan Channel through the Gulf of Mexico to its exit through the Florida Straits, it brings with it a large, deep mass of warmer water and strong currents . The LC’s position varies greatly from its retracted state in the Yucatan Channel, directly east of the Florida Straits, to its extended state into the far north and western Gulf. Although LCE has been studied for decades, accurate prediction of LCE shedding remains a challenging science problem that has a profound societal impact, for example, the rapid swirling ocean velocity associated with LC creates major safety concerns and disrupts operations of the 4000+ oil and gas drilling platforms in the Gulf. Preliminary study has shown that AI/ML approach is promising in predicting LCE. We will continue that effort by developing physically-based AI methods to improve both the long-term and short-term prediction and fundamental understanding of Loop Current Eddy shedding in the Gulf of Mexico.
3) User-inspired AI/ML research on ocean environment / Harmful Algal Blooms
Ranging from microscopic, single-celled organisms to large seaweeds, algae are simple plants that form the base of food webs. Under certain conditions, however, algae may grow out of control — and a few of these “blooms” produce toxins that can kill fish, mammals and birds, and may cause human illness or even death in extreme cases. Other algae are nontoxic, but eat up all of the oxygen in the water as they decay, clog the gills of fish and invertebrates, or smother corals and submerged aquatic vegetation. Still others discolor water, form huge, smelly piles on beaches or contaminate drinking water. Collectively, these events are called harmful algal blooms, or HABs. Every U.S. coastal and Great Lakes state experiences HABs. These annual occurring blooms are a national concern because they affect not only the health of people and marine ecosystems, but also the “health” of our economy — especially coastal communities dependent on the income of jobs generated through fishing and tourism. With climate change and increasing nutrient pollution, HABs can potentially occur more often and in locations not previously affected. We will develop physically based AI forecasts to predict both the general trend, and timing and locations of harmful algal blooms. Preliminary results demonstrated that ML can provide promising predictions and we will link both physically-based ML and robust training approaches to couple the AI methods with marine physical-ecosystem models to provide trustworthy HAB prediction.
Results
Work is in progress.
See the official project web site.